In the realm of artificial intelligence, a new and significant bottleneck is emerging that could hinder future advancements: the scarcity of high-quality data for training large language models (LLMs). This development comes at a time when computational power and energy resources, such as NVIDIA’s GPUs and electricity for data centers, are already under intense demand. The concern now shifts to the availability of data itself, as recent studies indicate a potential depletion of usable data as early as next year.
Table of Contents
The Growing Demand for Data
Historically, LLMs have relied heavily on vast amounts of human-generated text for training. Notable models like GPT-3, LLaMA 3, and Falcon have utilized approximately 300 trillion tokens of text data. However, projections suggest that if the current trend continues, the available data could be exhausted between 2026 and 2032, with some estimates indicating a potential shortage as early as 2025. This scenario raises critical questions about the future scalability of AI and the sustainability of current AI training methodologies.
Analysis of Data Availability
The research by Epoch AI highlights the limitations in the amount of human-generated text available for training LLMs. The current corpus of publicly accessible text data is estimated to be around 10^15 tokens, with potential variations based on the quality and nature of the data. Furthermore, even with conservative estimates, high-quality datasets that include curated content like academic papers and books are significantly smaller, approximately 10 trillion tokens.
Despite efforts to scrape more data from the web and incorporate diverse sources, the overall growth in available data does not keep pace with the increasing demands of AI models. This discrepancy suggests that by 2028, even the most optimized data utilization strategies could fall short, leading to a significant slowdown in AI development.
Innovations and Solutions
Interestingly, recent studies have shown that reusing existing datasets for multiple training epochs does not degrade model performance as previously thought. This finding opens up the possibility of making more efficient use of the available data, potentially extending the lifespan of current datasets. By training models on the same data multiple times, researchers can simulate the effect of having more data, thus mitigating the immediate impact of data scarcity.
The Role of Big Tech and Data Quality
As data becomes an increasingly valuable resource, companies with extensive, high-quality datasets are poised to play a crucial role in the AI landscape. Tech giants like Google, Meta, and Apple, which possess vast amounts of proprietary data, could gain a significant advantage. These companies can leverage their data assets to train more advanced AI models, maintaining their competitive edge even as public data sources dwindle.
Moreover, the emphasis will shift towards the quality of data rather than quantity alone. Effective data curation and the development of algorithms to select and refine training data will become essential. Ensuring that models are trained on the most relevant and high-quality data will be critical for advancing AI capabilities without exponentially increasing the data requirements.
Conclusion
The AI community faces a pivotal challenge: overcoming the impending data shortage that threatens to stall progress. While improvements in computational efficiency and data reuse strategies offer some relief, the long-term solution lies in better data management and leveraging the substantial data reserves held by major tech companies. As the landscape evolves, the ability to efficiently utilize and refine available data will determine the future trajectory of AI development.
By addressing these challenges head-on, the AI industry can continue to innovate and push the boundaries of what is possible, even in the face of growing resource constraints.
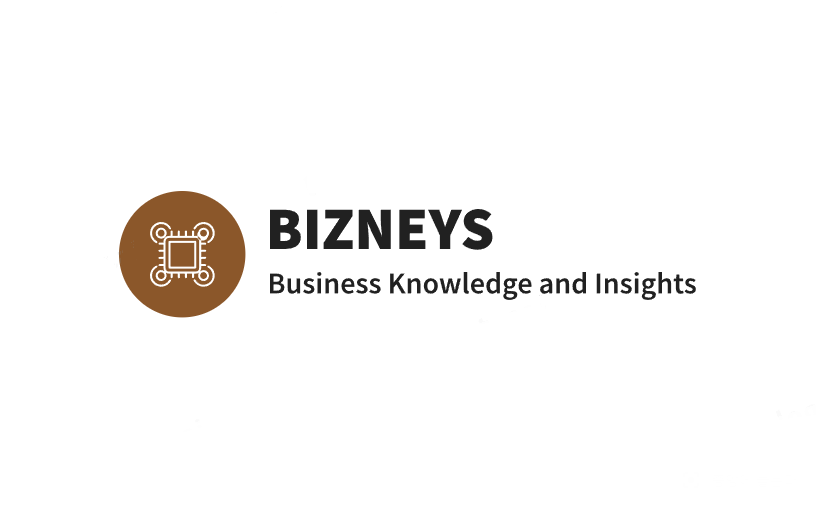